

At inference time, we merely add an optimality bias that will increase the chance of producing actions which can be related to larger returns.
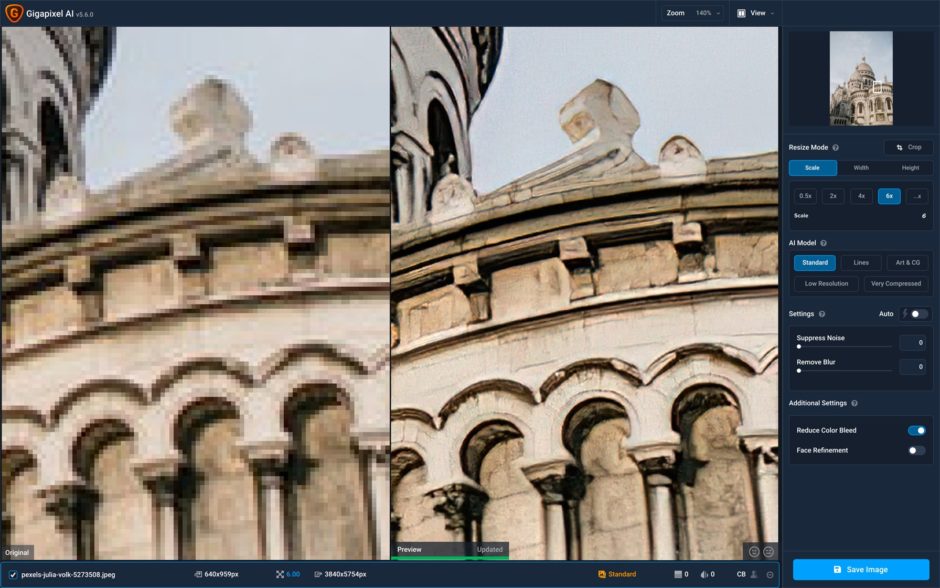
To deal with this situation, we as a substitute mannequin a distribution of return magnitudes primarily based on previous interactions with the surroundings throughout coaching. However, how have you learnt if a return is each optimum and stably achievable in a given surroundings? Earlier functions of Resolution Transformers relied on custom-made definitions of the specified return for every particular person job, which required manually defining a believable and informative vary of scalar values which can be appropriately interpretable alerts for every particular recreation - a job that’s non-trivial and slightly unscalable. So throughout inference, the Resolution Transformer can obtain any return worth within the vary it has seen throughout coaching, together with the optimum return.
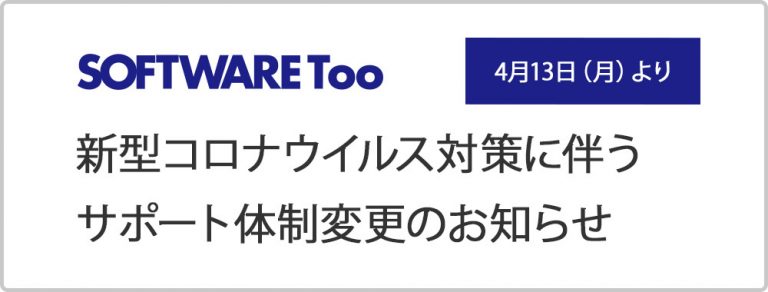
The concept is that coaching an agent on a variety of experiences (from newbie to knowledgeable degree) exposes the mannequin to a wider vary of variations in gameplay, which in flip helps it extract helpful guidelines of gameplay that enable it to succeed underneath any circumstance. As a substitute of studying a coverage to attain excessive return magnitude as in conventional reinforcement studying, Resolution Transformers map numerous experiences, starting from expert-level to beginner-level, to their corresponding return magnitude throughout coaching. A Resolution Transformer is a sequence mannequin that predicts future actions by contemplating previous interactions between an agent and the encircling surroundings, and (most significantly) a desired return to be achieved in future interactions. On this work, we use Decision Transformers as our spine method to coaching an RL agent. At each time step, an agent observes the surroundings (some additionally think about the interactions that occurred prior to now) and decides what motion to take to assist itself obtain the next return magnitude in future interactions. Conventional deep reinforcement studying brokers ( DQN, SimPLe, Dreamer, and many others) are skilled to optimize choices to attain the optimum return. This method considerably improves upon the few present options to studying multi-game brokers, corresponding to temporal difference (TD) studying or behavioral cloning (BC).Ī Multi-Sport Resolution Transformer (MGDT) can play a number of video games at desired degree of competency from coaching on a variety of trajectories spanning all ranges of experience.ĭon’t Optimize for Return, Simply Ask for Optimality In reinforcement studying, reward refers back to the incentive alerts which can be related to finishing a job, and return refers to cumulative rewards in a course of interactions between an agent and its surrounding surroundings. Our mannequin trains an agent that may play 41 Atari video games concurrently at close-to-human efficiency and that can be shortly tailored to new video games through fine-tuning. It’s pure to surprise, can an analogous technique be utilized in constructing generalist brokers for sequential resolution making? Can such fashions additionally allow quick adaptation to new duties, much like PaLM and Flamingo?Īs an preliminary step to reply these questions, in our current paper “ Multi-Game Decision Transformers” we discover the way to construct a generalist agent to play many video video games concurrently. Trying throughout current progress within the fields of pure language processing, imaginative and prescient, and generative fashions (corresponding to PaLM, Imagen, and Flamingo), we see that breakthroughs in making general-purpose fashions are sometimes achieved by scaling up Transformer-based fashions and coaching them on massive and semantically numerous datasets. Nonetheless, little progress has been made to increase these outcomes to generalist brokers that may not solely be able to performing many various duties, but in addition upon quite a lot of environments with probably distinct embodiments. Present deep reinforcement learning (RL) strategies can prepare specialist artificial agents that excel at decision-making on numerous particular person duties in particular environments, corresponding to Go or StarCraft.
#Luminar neo vs ai software
Posted by Winnie Xu, Scholar Researcher and Kuang-Huei Lee, Software program Engineer, Google Analysis, Mind Crew
